Data manipulation with Pandas
Contents
3.2. Data manipulation with Pandas#
3.2.1. What is Pandas?#
No, it is not the plural of panda the bear! Pandas is a Python package designed for data manipulation and analysis. Its name is derived from “panel data” (not the bear!) and is also from “Python data analysis” 1. It is especially popular for its data structures that help process tabular data in a fast and efficient way.
3.2.2. Importing Pandas#
To be able to work with Pandas we need to import the Pandas package into our Python code. Below is the community agreed convention:
import pandas as pd
3.2.3. The dataset#
For this session we will be using data from the World Bank Data Catalog 2 from the World Development Indicators (WDI) collection which was transformed by Alexia Cardona for the purpose of teaching data manipulation and visualisation programming. This data is compiled using official international resources.
Download the dataset from here and save it into a data
folder in your project.
This dataset contains official data for each country worldwide and contains indicators on life expectancy, population, and CO2 emissions. Below is the metadata of the dataset:
Column name |
Description |
---|---|
|
Name of country. |
|
Year data is representative of. |
|
Life expectancy at birth, female (years). Life expectancy at birth indicates the number of years a newborn infant would live if prevailing patterns of mortality at the time of its birth were to stay the same throughout its life. |
|
Life expectancy at birth, male (years). |
|
Life expectancy at birth, total (years). |
|
Land area (sq. km). |
|
Male population count. |
|
Female population count. |
|
Total population count. |
3.2.4. Reading tabular data from a file#
Now that we have everything in place, let us start by first reading our data into our Python code. Pandas has conventient functions that load files containing tabular data.
df = pd.read_csv("data/world-bank-1_data.csv")
The code above reads the file world-bank-1_data.csv
into a DataFrame
object and creates variable df
that points to it.
There are other functions in Pandas that read other file formats. You can see a list of these here.
3.2.5. The DataFrame#
The popularity of Pandas mainly lies with its data structure; the DataFrame
. The DataFrame data structure is suitable for
data that is in tabular form (2-dimensional); data with columns and rows. Since most of the data comes into this form, it makes the DataFrame data structure
and Pandas widely used.
Now that we have loaded the DataFrame in Python, we need to check if the data has loaded properly. Let us try to check this in the Console, by printing the first 5 rows of the DataFrame:
df.head(5)
country year population_m population_f population_t \
0 Afghanistan 2000 10689508.0 10090449.0 20779957.0
1 Afghanistan 2001 11117754.0 10489238.0 21606992.0
2 Afghanistan 2002 11642106.0 10958668.0 22600774.0
3 Afghanistan 2003 12214634.0 11466237.0 23680871.0
4 Afghanistan 2004 12763726.0 11962963.0 24726689.0
population_density land_area life_expectancy_f life_expectancy_m \
0 31.859861 652230.0 57.120 54.663
1 33.127872 652230.0 57.596 55.119
2 34.651540 652230.0 58.080 55.583
3 36.307546 652230.0 58.578 56.056
4 37.910996 652230.0 59.093 56.542
life_expectancy_t co2_emissions_pc
0 55.841 0.036574
1 56.308 0.033785
2 56.784 0.045574
3 57.271 0.051518
4 57.772 0.041655
You can also get summary statistics for each column of the df
DataFrame:
df.describe()
year population_m population_f population_t \
count 3038.000000 2.708000e+03 2.708000e+03 3.030000e+03
mean 2010.500000 1.810384e+07 1.781787e+07 3.211690e+07
std 7.229606 6.969846e+07 6.568821e+07 1.284477e+08
min 2000.000000 3.571000e+04 4.029700e+04 9.392000e+03
25% 2003.000000 9.764312e+05 9.934100e+05 6.599435e+05
50% 2013.500000 3.641720e+06 3.747408e+06 5.644552e+06
75% 2017.000000 1.234708e+07 1.228930e+07 1.980094e+07
max 2020.000000 7.237710e+08 6.873290e+08 1.411100e+09
population_density land_area life_expectancy_f life_expectancy_m \
count 2968.000000 2.998000e+03 2800.000000 2800.000000
mean 410.489783 6.063802e+05 72.870253 67.979873
std 1894.724659 1.755858e+06 9.485278 8.831021
min 0.136492 2.027000e+00 40.005000 38.861000
25% 32.530300 1.014750e+04 67.318000 62.900000
50% 83.018459 9.428000e+04 75.450000 69.591000
75% 207.487240 4.512200e+05 79.900000 74.555250
max 21388.600000 1.638139e+07 88.100000 82.900000
life_expectancy_t co2_emissions_pc
count 2800.000000 2481.000000
mean 70.379877 4.294507
std 9.085448 5.431735
min 39.441000 0.020367
25% 65.076000 0.651679
50% 72.409000 2.463436
75% 77.124707 6.080600
max 85.387805 50.954034
You can also get a summary of the dtype
of each column together with the number of non-null values for each column.
df.info()
<class 'pandas.core.frame.DataFrame'>
RangeIndex: 3038 entries, 0 to 3037
Data columns (total 11 columns):
# Column Non-Null Count Dtype
--- ------ -------------- -----
0 country 3038 non-null object
1 year 3038 non-null int64
2 population_m 2708 non-null float64
3 population_f 2708 non-null float64
4 population_t 3030 non-null float64
5 population_density 2968 non-null float64
6 land_area 2998 non-null float64
7 life_expectancy_f 2800 non-null float64
8 life_expectancy_m 2800 non-null float64
9 life_expectancy_t 2800 non-null float64
10 co2_emissions_pc 2481 non-null float64
dtypes: float64(9), int64(1), object(1)
memory usage: 261.2+ KB
None
Alternatively you can get the dtype
of each column by using the dtypes
attribute of the DataFrame; df.dtypes
. Note that
columns which hold string data values are imported into Pandas as object
dtype
.
To get the number of rows and columns that the DataFrame is made up of use the shape
attribute:
df.shape
(3038, 11)
Note that shape
is an attribute not a method, so when called, no parentheses are used after shape
.
3.2.6. The Series#
Each column in a DataFrame is a Series. A Series is a data structure in Pandas and is essentially a 1-dimensional array. Fig. 3.2 shows the main components of the Series.
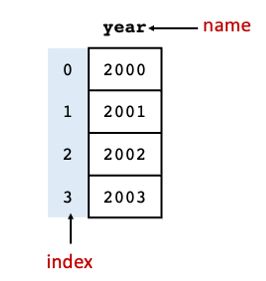
Fig. 3.2 Components of the Series data structure.#
Exercise 3.4 (Exploring DataFrames)
Level:
In this exercise we will be using the dataset from the World Bank Data Catalog that is used in this course. Follow the instructions above to load the dataset into Python and make use of DataFrame methods to answer the following questions:
What type is
df
?How many columns and how many rows does the
df
have?What are the data types of each column?
3.2.7. Slicing DataFrames#
Fig. 3.3 shows the core components of the DataFrame data structure. The first column we see when we look at the
contents of df
is the index. The index is a column of data items in the DataFrame but rather it contains the index
positions of each record in the DataFrame. Like all the other data structures in Python we have looked at so far, it
starts with 0.
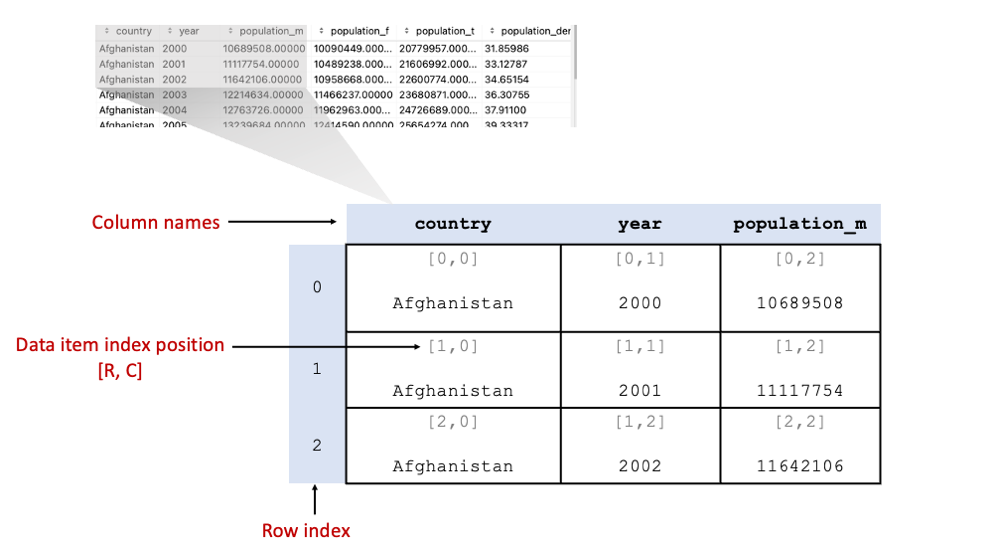
Fig. 3.3 Components of the DataFrame data structure.#
We can extract columns, rows or data items from DataFrames in different ways. The sections below go over the different ways we can slice DataFrames.
3.2.7.1. Extracting column data (label-based indexing)#
To extract data from a column in the DataFrame we use the following notation:
df[“column_name”]
where df
is the DataFrame object. If we want to extract multiple columns, we can provide a Python list of column names
inside the square brackets as follows:
df[[“column_name1”, “column_name2”]]
#extract the column "life_expectancy_t" and save it in variable le_t
le_t = df["life_expectancy_t"]
#check what type is le_t
print(f"Type of le_t is: {type(le_t)}")
# get the length of the Series
print(f"Size of le_t is: {le_t.size}")
#print the first 5 items
le_t.head()
Type of le_t is: <class 'pandas.core.series.Series'>
Size of le_t is: 3038
0 55.841
1 56.308
2 56.784
3 57.271
4 57.772
Name: life_expectancy_t, dtype: float64
Note that in the example above, since we are extracting only one column from df
the result of the first slicing is a Series object.
The code below, on the other hand, extracts 3 columns from df
, and therefore the result of this is another DataFrame object.
#extract the columns "life_expectancy_t", "life_expectancy_m", "life_expectancy_f" and save it in variable le
le = df[["life_expectancy_t", "life_expectancy_m", "life_expectancy_f"]]
# get type of object returned from slicing
print(f"Type of le is: {type(le)}")
#get size of DataFrame
print(f"Size of le is: {le.shape}")
# print the first 5 items
le.head()
Type of le is: <class 'pandas.core.frame.DataFrame'>
Size of le is: (3038, 3)
life_expectancy_t | life_expectancy_m | life_expectancy_f | |
---|---|---|---|
0 | 55.841 | 54.663 | 57.120 |
1 | 56.308 | 55.119 | 57.596 |
2 | 56.784 | 55.583 | 58.080 |
3 | 57.271 | 56.056 | 58.578 |
4 | 57.772 | 56.542 | 59.093 |
3.2.7.2. Extracting row data (boolean indexing)#
We can extract rows from our DataFrame that meet a specified condition by using the conditional expressions in our slice operators as follows:
df[conditional expression]
Let us try an example on our df
DataFrame. We would like to get the records where life expectancy is more than 80:
le_top = df[df["life_expectancy_t"] > 80]
le_top.head()
country | year | population_m | population_f | population_t | population_density | land_area | life_expectancy_f | life_expectancy_m | life_expectancy_t | co2_emissions_pc | |
---|---|---|---|---|---|---|---|---|---|---|---|
143 | Australia | 2003 | 9926226.0 | 9969174.0 | 19895400.0 | 2.589771 | 7682300.0 | 82.8 | 77.8 | 80.239024 | 17.721684 |
144 | Australia | 2004 | 10042276.0 | 10085124.0 | 20127400.0 | 2.619971 | 7682300.0 | 83.0 | 78.1 | 80.490244 | 18.174727 |
145 | Australia | 2005 | 10177992.0 | 10216808.0 | 20394800.0 | 2.654778 | 7682300.0 | 83.3 | 78.5 | 80.841463 | 18.146292 |
146 | Australia | 2013 | 11541758.0 | 11586371.0 | 23128129.0 | 3.010574 | 7682300.0 | 84.3 | 80.1 | 82.148780 | 16.442316 |
147 | Australia | 2014 | 11705167.0 | 11770519.0 | 23475686.0 | 3.055815 | 7682300.0 | 84.4 | 80.3 | 82.300000 | 15.830422 |
Similarly to Numpy, you can combine different comparison expressions using the &
(and) or |
(or) operators. You would
need to wrap each conditional expression in parentheses. See example below:
# get all records that have life expectancy > 80, where year is either 2020 or 2019
le_top[(le_top["year"] == 2020) | (le_top["year"] == 2019)]
country | year | population_m | population_f | population_t | population_density | land_area | life_expectancy_f | life_expectancy_m | life_expectancy_t | co2_emissions_pc | |
---|---|---|---|---|---|---|---|---|---|---|---|
152 | Australia | 2019 | 12632259.0 | 12733486.0 | 25365745.0 | 3.297670 | 7692020.000 | 85.0 | 80.9 | 82.900000 | 15.238267 |
153 | Australia | 2020 | 12794929.0 | 12898338.0 | 25693267.0 | 3.340250 | 7692020.000 | 85.3 | 81.2 | 83.200000 | NaN |
166 | Austria | 2019 | 4372358.0 | 4507562.0 | 8879920.0 | 107.609307 | 82520.000 | 84.2 | 79.7 | 81.895122 | 7.293984 |
167 | Austria | 2020 | 4395554.0 | 4521310.0 | 8916864.0 | 108.057004 | 82520.000 | 83.6 | 78.9 | 81.192683 | NaN |
264 | Belgium | 2019 | 5686560.0 | 5802420.0 | 11488980.0 | 379.424703 | 30280.000 | 84.3 | 79.8 | 81.995122 | 8.095584 |
... | ... | ... | ... | ... | ... | ... | ... | ... | ... | ... | ... |
2631 | Sweden | 2020 | 5186267.0 | 5167175.0 | 10353442.0 | 25.420723 | 407283.532 | 84.2 | 80.7 | 82.407317 | NaN |
2644 | Switzerland | 2019 | 4252686.0 | 4322594.0 | 8575280.0 | 217.007630 | 39516.030 | 85.8 | 82.1 | 83.904878 | 4.359041 |
2645 | Switzerland | 2020 | 4284690.0 | 4351871.0 | 8636561.0 | 218.558418 | 39516.030 | 85.2 | 81.1 | 83.100000 | NaN |
2882 | United Kingdom | 2019 | 33008768.0 | 33827559.0 | 66836327.0 | 276.263080 | 241930.000 | 83.1 | 79.4 | 81.204878 | 5.220514 |
2883 | United Kingdom | 2020 | 33144663.0 | 33936337.0 | 67081000.0 | 277.274418 | 241930.000 | 82.9 | 79.0 | 80.902439 | NaN |
78 rows × 11 columns
You can write the same code using the isin()
function which providing it with a list of values, it returns True
for
each row where the values are present. The example below returns the same result as the code above, but it uses the isin()
function to check whether each row in le_top
has either 2000
or 2019
in the year
column.
le_top[le_top["year"].isin([2020, 2019])]
country | year | population_m | population_f | population_t | population_density | land_area | life_expectancy_f | life_expectancy_m | life_expectancy_t | co2_emissions_pc | |
---|---|---|---|---|---|---|---|---|---|---|---|
152 | Australia | 2019 | 12632259.0 | 12733486.0 | 25365745.0 | 3.297670 | 7692020.000 | 85.0 | 80.9 | 82.900000 | 15.238267 |
153 | Australia | 2020 | 12794929.0 | 12898338.0 | 25693267.0 | 3.340250 | 7692020.000 | 85.3 | 81.2 | 83.200000 | NaN |
166 | Austria | 2019 | 4372358.0 | 4507562.0 | 8879920.0 | 107.609307 | 82520.000 | 84.2 | 79.7 | 81.895122 | 7.293984 |
167 | Austria | 2020 | 4395554.0 | 4521310.0 | 8916864.0 | 108.057004 | 82520.000 | 83.6 | 78.9 | 81.192683 | NaN |
264 | Belgium | 2019 | 5686560.0 | 5802420.0 | 11488980.0 | 379.424703 | 30280.000 | 84.3 | 79.8 | 81.995122 | 8.095584 |
... | ... | ... | ... | ... | ... | ... | ... | ... | ... | ... | ... |
2631 | Sweden | 2020 | 5186267.0 | 5167175.0 | 10353442.0 | 25.420723 | 407283.532 | 84.2 | 80.7 | 82.407317 | NaN |
2644 | Switzerland | 2019 | 4252686.0 | 4322594.0 | 8575280.0 | 217.007630 | 39516.030 | 85.8 | 82.1 | 83.904878 | 4.359041 |
2645 | Switzerland | 2020 | 4284690.0 | 4351871.0 | 8636561.0 | 218.558418 | 39516.030 | 85.2 | 81.1 | 83.100000 | NaN |
2882 | United Kingdom | 2019 | 33008768.0 | 33827559.0 | 66836327.0 | 276.263080 | 241930.000 | 83.1 | 79.4 | 81.204878 | 5.220514 |
2883 | United Kingdom | 2020 | 33144663.0 | 33936337.0 | 67081000.0 | 277.274418 | 241930.000 | 82.9 | 79.0 | 80.902439 | NaN |
78 rows × 11 columns
3.2.7.3. Extracting rows and columns#
To extract rows and columns from a DataFrame we need to use the loc
and iloc
operators.
3.2.7.3.1. loc
#
loc
is mainly used with label-based indexing and boolean indexing. The general usage format is the following:
df.loc[row condition expression, column_name]
In the example below we extract the list of countries from our df
DataFrame where life expectancy is greater than 80.
countries = df.loc[df["life_expectancy_t"] > 80, "country"]
print(f"Type of countries is: {type(countries)}")
print(f"Length of Series is: {countries.size}")
# use unique method of Series to remove duplicates
print(countries.unique())
Type of countries is: <class 'pandas.core.series.Series'>
Length of Series is: 333
['Australia' 'Austria' 'Belgium' 'Bermuda' 'Canada' 'Channel Islands'
'Chile' 'Costa Rica' 'Cyprus' 'Denmark' 'Faroe Islands' 'Finland'
'France' 'Germany' 'Greece' 'Guam' 'Hong Kong SAR, China' 'Iceland'
'Ireland' 'Israel' 'Italy' 'Japan' 'Korea, Rep.' 'Liechtenstein'
'Luxembourg' 'Macao SAR, China' 'Malta' 'Netherlands' 'New Zealand'
'Norway' 'Portugal' 'Puerto Rico' 'Qatar' 'Singapore' 'Slovenia' 'Spain'
'St. Martin (French part)' 'Sweden' 'Switzerland' 'United Kingdom']
3.2.7.3.2. iloc
(integer-based indexing)#
iloc
is mainly used with integer-based indexing, which means that index positions are supplied to iloc
in the following
format:
df[R, C]
where R is the row index position and C is the column index position. In short, if you want to extract data items from a
DataFrame based on index positions, than iloc
is the way to do that.
#get the first 10 rows and the first 5 columns of the df Dataframe
df.iloc[0:10, 0:5]
country | year | population_m | population_f | population_t | |
---|---|---|---|---|---|
0 | Afghanistan | 2000 | 10689508.0 | 10090449.0 | 20779957.0 |
1 | Afghanistan | 2001 | 11117754.0 | 10489238.0 | 21606992.0 |
2 | Afghanistan | 2002 | 11642106.0 | 10958668.0 | 22600774.0 |
3 | Afghanistan | 2003 | 12214634.0 | 11466237.0 | 23680871.0 |
4 | Afghanistan | 2004 | 12763726.0 | 11962963.0 | 24726689.0 |
5 | Afghanistan | 2005 | 13239684.0 | 12414590.0 | 25654274.0 |
6 | Afghanistan | 2013 | 16554278.0 | 15715314.0 | 32269592.0 |
7 | Afghanistan | 2014 | 17138803.0 | 16232001.0 | 33370804.0 |
8 | Afghanistan | 2015 | 17686166.0 | 16727437.0 | 34413603.0 |
9 | Afghanistan | 2016 | 18186994.0 | 17196034.0 | 35383028.0 |
Exercise 3.5 (Slicing DataFrames)
Level:
Using the df
DataFrame created above, write code to perform the following tasks:
Get the first 3 columns of
df
.Get the first 3 rows of
df
.Get the first row of
df
.What are the maximum and minimum values of life expectancy (use life_expectancy_t column)? Which countries have these?
Extract all the data from the United Kingdom and save it into another DataFrame. How many records were returned?
Extract all the records from the United Kingdom from the year 2020. How many records were returned?
When extracting rows from a DataFrame, try not using parentheses between two conditional expressions when using
&
or|
and see what happens.